ChatGPT Sentiment Analysis Example: Understanding Sentiment Analysis in ChatGPT Models
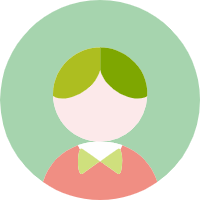
ChatGPT Sentiment Analysis Example: A Comprehensive Guide to Sentiment Analysis with ChatGPT
Sentiment analysis is a critical natural language processing (NLP) task that involves determining the polarity (positive, negative, or neutral) of textual data. With the rapid development of artificial intelligence (AI) and machine learning, sentiment analysis has become increasingly important in various industries, such as customer service, marketing, and social media monitoring. In this article, we will explore a comprehensive guide to using ChatGPT, a powerful AI language model, for sentiment analysis.
1. Understanding Sentiment Analysis
Sentiment analysis aims to classify textual data into predefined categories, such as positive, negative, or neutral, based on the emotional content of the text. This can be used for various purposes, such as analyzing customer feedback, monitoring brand reputation, or even predicting market trends.
2. ChatGPT Overview
ChatGPT is an advanced AI language model developed by OpenAI, which has gained significant attention for its ability to generate human-like text and interact with users. By leveraging the power of ChatGPT, we can create sophisticated sentiment analysis models that can understand and analyze the sentiment behind text data.
3. Preparing Data for Sentiment Analysis
Before using ChatGPT for sentiment analysis, it is essential to prepare the data. This includes cleaning the text data, preprocessing it, and ensuring that it is suitable for analysis. Some important steps in this process include:
- Removing punctuation and special characters
- Converting text to lowercase
- Removal of stopwords, such as "the," "and," "in," etc.
- Normalizing text by applying a specific syntax and grammar
4. Training a Sentiment Analysis Model with ChatGPT
Once the data is prepared, it is time to train a sentiment analysis model with ChatGPT. The following steps will help you achieve this:
- Generate input text using ChatGPT and its natural language understanding capability
- Preprocess the input text as described in Step 3
- Feed the preprocessed text to ChatGPT and request it to generate output, which can be a sentiment label (positive, negative, or neutral) or a probability distribution over the three categories
- Train a classifier (e.g., logistic regression, support vector machine, or a deep learning model) using the output from ChatGPT and the original sentiment labels
- Evaluate the performance of the classifier on a test dataset and optimize it as necessary
5. Validating and Deploying the Sentiment Analysis Model
Once the sentiment analysis model is trained and optimized, it is essential to validate its performance and deploy it for use. Some important steps in this process include:
- Evaluate the model's performance on a separate test dataset to ensure that it generalizes well and does not overfit
- Use the model for sentiment analysis on real-world text data, such as customer reviews, social media posts, or product descriptions
- Monitor the model's performance in real-time and make necessary adjustments to optimize its accuracy and efficiency
In conclusion, ChatGPT, an advanced AI language model, offers a unique opportunity to explore sentiment analysis with its powerful natural language understanding capability. By following a comprehensive guide to preparing data, training a sentiment analysis model, and validating and deploying it, one can create sophisticated sentiment analysis models that can help businesses and organizations understand and analyze the sentiment behind text data, leading to better decision-making and improved outcomes.