Social media sentiment analysis tools: Tools and Techniques for Analyzing Social Media Sentiment
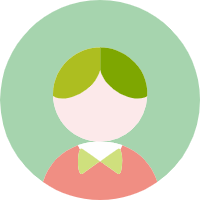
Social media has become an essential tool for businesses, brands, and individuals to engage with their audience, collect feedback, and make informed decisions. One of the most significant challenges in social media management is understanding and analyzing the sentiment expressed by users. Sentiment analysis, also known as opinion mining, is a technique that helps businesses and marketers gauge the sentiment of users towards a product, service, or brand. This article will discuss the various tools and techniques used in social media sentiment analysis, their benefits, and limitations.
Tools for Social Media Sentiment Analysis
1. Traditional Text Analysis Techniques
Traditional text analysis techniques, such as keyword counting and sentiment dictionaries, can be used to analyze the sentiment expressed in social media posts. Keyword counting involves identifying and counting words or phrases that are considered positive, negative, or neutral in a given text. Sentiment dictionaries, on the other hand, are pre-defined collections of words and phrases that are associated with a particular sentiment (e.g., positive, negative, or neutral). These techniques are simple to implement but may lack the accuracy and depth required for complex sentiment analysis tasks.
2. Machine Learning-based Methods
Machine learning-based methods, such as support vector machines (SVM), decision trees, and natural language processing (NLP) algorithms, have become more popular in social media sentiment analysis. These methods can automatically learn patterns and identify sentiment from large volumes of text data. However, they require significant training data and computational resources, which can be time-consuming and expensive.
3. Deep Learning Techniques
Deep learning techniques, such as recurrent neural networks (RNN) and long short-term memory (LSTM) models, have gained traction in recent years due to their ability to capture complex patterns and relationships in text data. These models can automatically learn the sentiment of a given text by analyzing the sequence of words and phrases. Deep learning techniques have shown great potential in sentiment analysis, but they require significant computational resources and may struggle with the complexity and diversity of social media data.
4. Pre-trained Language Models
Pre-trained language models, such as BERT, GPT, and RoBERT, have become popular in sentiment analysis due to their ability to capture rich context and semantics in text data. These models are pre-trained on large corpus of text data and can be fine-tuned for specific sentiment analysis tasks. Pre-trained language models have shown great accuracy and efficiency in sentiment analysis, but they may require significant time and resources for fine-tuning and model training.
Benefits of Social Media Sentiment Analysis Tools
1. Enhanced Decision-making: Sentiment analysis tools can help businesses and marketers gain insights into the sentiment of users towards their products, services, or brands, enabling informed decision-making.
2. Improved Customer Service: Understanding customer sentiment can help businesses and brands improve their customer service by addressing the concerns and issues raised by users.
3. Product and Service Improvement: Sentiment analysis can help businesses and brands identify areas where their products or services may be lacking, leading to improvements in product and service quality.
4. Competitive Advantage: Gaining insights into the sentiment of users towards competitors can help businesses and brands stay ahead of the competition and adapt to market trends.
Limitations of Social Media Sentiment Analysis Tools
1. Data Quality: The quality of social media data can be variable, with posts containing inappropriate, inaccurate, or biased information. This can affect the accuracy and reliability of sentiment analysis tools.
2. Data Privacy: Analyzing social media data can be a sensitive process, with concerns about data privacy and user privacy rights. Businesses and marketers must ensure that they have valid consent and comply with data protection regulations.
3. Time and Resource Consumption: Sentiment analysis tools, particularly those based on machine learning or deep learning techniques, can consume significant time and resources for model training, fine-tuning, and data processing.
4. Lacking Accuracy: Despite advancements in machine learning and deep learning techniques, sentiment analysis tools may struggle with accuracy, particularly in complex and diverse social media data.
Social media sentiment analysis tools offer valuable insights into the sentiment of users towards products, services, or brands, enabling informed decision-making and improved customer service. However, these tools are not without limitations, and businesses and marketers must carefully consider the benefits and drawbacks of using these tools in their social media management strategies. By understanding the advantages and disadvantages of various tools and techniques, businesses and brands can make informed choices when implementing sentiment analysis tools for their social media management.