stock algorithmic trading strategies: Algorithmic Trading Strategies for Stocks
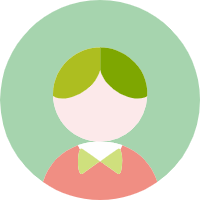
Stock Algorithmic Trading Strategies: Effective Techniques for the Modern Trader
The world of trading has undergone a significant transformation in recent years. With the advent of electronic trading platforms and the rapid development of technology, algorithmic trading has become an increasingly important aspect of the financial markets. Algorithmic trading, also known as automated trading, refers to the use of computer programs to execute trades at high speeds and with minimal human intervention. In this article, we will explore some of the key algorithmic trading strategies for stocks, their benefits, and how to implement them effectively.
1. Rule-based trading strategies
Rule-based trading strategies involve the use of pre-defined rules to guide the execution of trades. These rules can be based on various factors, such as price, volume, technical indicators, or fundamental data. One popular rule-based trading strategy is the use of moving averages, which can help identify potential trend changes and potential entry and exit points for trades.
2. Model-based trading strategies
Model-based trading strategies involve the use of mathematical models to predict the likelihood of future price movements. These models can be based on various methods, such as machine learning, statistics, or econometrics. One popular model-based trading strategy is the use of neural networks, which can help identify complex patterns and relationships in the stock market data.
3. Data-driven trading strategies
Data-driven trading strategies involve the use of large volumes of historical and real-time market data to identify patterns and trends. These strategies often rely on machine learning algorithms to analyze the data and generate trade suggestions. One popular data-driven trading strategy is the use of machine learning algorithms, such as support vector machines or decision trees, to predict future price movements based on historical data.
4. Signal-based trading strategies
Signal-based trading strategies involve the use of external data sources, such as news, economic data, or social media, to generate trade signals. These signals can be used to guide the execution of trades at specific points in the stock market cycle. One popular signal-based trading strategy is the use of social sentiment analytics, which can help identify potential market shifts based on public sentiment towards specific stocks or market sectors.
Implementing Algorithmic Trading Strategies
In order to successfully implement algorithmic trading strategies, traders must consider the following factors:
1. Understanding the strategy: Before implementing any trading strategy, it is essential to fully understand the strategy's principles, benefits, and potential risks.
2. Selecting the right platform: There are many electronic trading platforms available, each with its own set of features and limitations. Traders should select a platform that best suits their trading strategy and requirements.
3. Testing and fine-tuning: It is essential to test and fine-tune the trading strategy on a demo account before implementing it on a live account. This will help identify potential issues and optimize the strategy for better performance.
4. Risk management: When implementing algorithmic trading strategies, it is crucial to implement robust risk management measures, such as stop-loss orders and risk caps, to protect against potential losses.
5. Continuous improvement: The stock market is a dynamic environment, and trading strategies must be continuously reviewed and updated to stay relevant and effective.
Algorithmic trading strategies offer a powerful tool for modern traders to maximize efficiency, reduce emotional bias, and improve trading performance. By understanding and implementing the right strategies, traders can harness the power of technology to create successful and profitable trading experiences.