Token Passing: Understanding the Advantages and Disadvantages of Token Passing in Networks
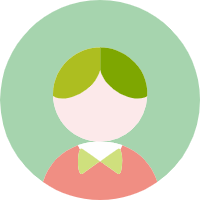
Token passing is a widely used concept in the field of network science, particularly in the study of social networks. It is a model that represents the exchange of information between nodes in a network, where each node represents a person or entity. Token passing helps researchers better understand the dynamics of information spread and the impact of network structure on the spread of ideas, beliefs, and behaviors. This article aims to provide an overview of token passing, its advantages, and disadvantages, as well as its applications in real-world networks.
Advantages of Token Passing
1. Simplifies complex interactions: Token passing can help simplify the understanding of complex social networks by representing the exchange of information between nodes using a simple token system. This can make it easier for researchers to visualize and analyze the network structure.
2. Captures the dynamics of information spread: Token passing models can capture the dynamic nature of information spread in social networks by considering the time it takes for information to be passed from one node to another. This can help researchers better understand the rate at which ideas and behaviors are spread through a network.
3. Explores the impact of network structure: Token passing models can explore the impact of network structure on the spread of information by considering the connections between nodes and the potential for information to be passed between them. This can help researchers better understand the role of network structure in the spread of ideas and behaviors.
4. Quantifies the influence of individual nodes: Token passing models can help quantify the influence of individual nodes in a network by considering the number of tokens they hold and the potential for information to be passed to other nodes. This can help researchers better understand the role of individual nodes in the spread of information and the potential for their actions to have significant consequences.
Disadvantages of Token Passing
1. May be overly simplified: While token passing can help simplify the understanding of complex social networks, it may be overly simplified and lack the complexity found in real-world networks. This can lead to inaccurate predictions and understandings of the dynamics of information spread in these networks.
2. May ignore relevant factors: Token passing models may ignore important factors such as trust, social ties, and personal relationships that can impact the spread of information in a network. This can lead to an underestimation of the role these factors play in the dynamics of information spread.
3. Difficult to generalize: Token passing models can be challenging to generalize, as they may not be applicable to all types of social networks or information spread dynamics. This can make it difficult for researchers to apply the findings of token passing models to different network structures and scenarios.
Applications of Token Passing in Real-World Networks
Token passing has been applied to a wide range of real-world networks, including social networks, online communities, and communication networks. In social networks, token passing models have been used to explore the spread of ideas, beliefs, and behaviors, as well as the impact of network structure on the spread of information. In online communities, token passing models have been used to understand the dynamics of information spread and the role of individual nodes in the generation of content. In communication networks, token passing models have been used to explore the impact of network structure on the efficiency of information spread and the potential for errors and congestion.
Token passing is a useful tool in the study of social networks and the spread of information, but it should not be seen as a panacea for understanding all aspects of network dynamics. By considering the advantages and disadvantages of token passing, researchers can better understand the limitations of this model and explore alternative methods to capture the complexity of real-world networks. Ultimately, the most effective approach to understanding the dynamics of information spread in networks is likely a combination of different models and techniques, taking into account the unique characteristics of each network and the dynamic nature of information spread.