Variational Quantum Algorithms Review:A Comprehensive Overview of Variational Quantum Algorithms
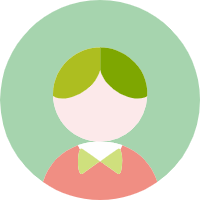
Variational Quantum Algorithms Review: A Comprehensive Overview and Assessment of Variational Quantum Algorithms
Variational quantum algorithms (VQA) have emerged as a promising approach to solve complex problems in quantum mechanics and machine learning. They combine the power of quantum computing with the efficiency of machine learning to create new ways to optimize and simulate complex systems. In this article, we provide a comprehensive overview of the VQA framework, its applications, and an assessment of its current state and future prospects.
1. Overview of Variational Quantum Algorithms
Variational quantum algorithms are based on the idea of using quantum states to approximate the solution of a problem. In this context, the quantum states are represented by so-called quantum circuits, which consist of quantum bits (qubits) and quantum gates. The goal of the algorithm is to find a quantum state that minimizes a specific cost function, which is related to the problem being solved. This process is known as the gradient descent method, and it allows the quantum state to evolve towards the optimal solution.
2. Applications of Variational Quantum Algorithms
Variational quantum algorithms have been applied to a wide range of problems in quantum mechanics, such as quantum chemistry, materials science, and artificial intelligence. Some of the most promising applications include:
a) Quantum Chemistry: VQA can be used to approximate the ground state energy of a molecular system, which is crucial for understanding the chemical properties of molecules and materials.
b) Materials Science: VQA can be used to predict the properties of new materials, such as electrical conductivity, thermal conductivity, and other important properties, which are crucial for the development of new technologies.
c) Artificial Intelligence: VQA can be used as a subroutine in machine learning algorithms, such as deep learning, to improve the accuracy and efficiency of the learning process.
3. Assessment of Variational Quantum Algorithms
Despite the promising results obtained with VQA, there are still several challenges and limitations that need to be addressed. Some of the main issues include:
a) Optimization: The optimization process in VQA is often computationally expensive, which can limit its applicability to large-scale problems.
b) Quantization: The quality of the approximation depends on the quality of the quantization of the problem, which can be challenging due to the complexity of the problem and the noise in the quantum hardware.
c) Scalability: VQA are generally not scalable, as the number of qubits in the quantum state grows exponentially with the complexity of the problem.
4. Future Prospects
Despite the challenges, the potential of VQA is immense, and several researchers and organizations are working on improving its performance and scalability. Future developments in quantum computing hardware, such as improved quantum error correction and reduced noise, are expected to significantly enhance the efficiency and accuracy of VQA. Furthermore, the integration of VQA with other machine learning techniques, such as variational autoencoders, is expected to open new possibilities for solving complex problems.
Variational quantum algorithms offer a promising approach to solve complex problems in quantum mechanics and machine learning. However, challenges such as optimization, quantization, and scalability need to be addressed to fully harness the potential of VQA. Future developments in quantum computing hardware and integration with other machine learning techniques are expected to further improve the performance and applicability of VQA, making them a powerful tool for solving complex problems in various fields.