market manipulation detection: A Systematic Literature Review of Market Manipulation Detection Methods
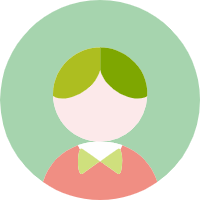
Market manipulation is an illegal practice in the financial market, where traders try to manipulate the price of a security to gain illegal benefits. Detection of market manipulation is crucial for maintaining the integrity of the financial market and protecting investors from potential harm. This article aims to provide a systematic review of the existing market manipulation detection methods, their strengths and weaknesses, and the potential future directions in this field.
Methodology
To conduct this review, a comprehensive search of the literature was conducted using various electronic databases, including Google Scholar, Microsoft Academic, and the Investment Research Database (IRD). The search terms included "market manipulation", "manipulation detection", "detection methods", "illegal trading", and "financial crime". The articles were selected based on their relevance to market manipulation detection and their methodological approach.
Existing Methods
The reviewed articles presented various methods for detecting market manipulation, which can be broadly classified into four categories:
1. Pattern-based methods: These methods rely on identifying unusual trading patterns in the market, which may indicate manipulation. Examples of pattern-based methods include Fourier transform, wavelet transform, and statistical tests such as autocorrelation and regression analysis.
2. Network-based methods: These methods focus on analyzing the relationship between traders and their trading activities, with the aim of identifying potential manipulation. Examples of network-based methods include social network analysis, community detection, and centrality measures.
3. Machine learning methods: These methods use mathematical models to identify patterns in the data and predict potential manipulation. Examples of machine learning methods include support vector machines, decision trees, and neural networks.
4. Textual analysis methods: These methods focus on analyzing the text of market communications, such as news articles, social media posts, and trading alerts, to identify potential manipulation. Examples of textual analysis methods include sentiment analysis, topic modeling, and natural language processing.
Evaluation of Existing Methods
The reviewed articles reported mixed results on the effectiveness of the existing market manipulation detection methods. Some methods showed promising results in identifying potential manipulation, while others were found to be insufficient or even misleading. The success of a method depends on several factors, including the type of manipulation, the complexity of the market, and the availability of relevant data.
Future Directions
Based on the findings of this review, several potential future directions in market manipulation detection were identified:
1. Integrating multiple methods: Combining multiple methods may improve the accuracy and reliability of market manipulation detection. This could involve a hybrid approach, where different methods are used to complement each other and provide a more comprehensive assessment of potential manipulation.
2. Leveraging big data: The increasing availability of big data in the financial market presents a valuable resource for market manipulation detection. Methods that can effectively utilize this data to identify potential manipulation are likely to be more effective.
3. Addressing ethical concerns: The use of data-driven methods in market manipulation detection raises ethical concerns, such as privacy issues and potential bias in the data. Future research should address these concerns by developing methods that are transparent, fair, and in line with regulatory requirements.
4. Exploring new methods: As the complexity of the financial market grows, it is crucial to explore new methods that can adapt to the changing environment and provide more accurate and reliable detection of market manipulation.
Market manipulation detection is a complex and challenging task, and the existing methods have their own limitations. Future research should focus on developing hybrid methods that can effectively utilize multiple sources of data and address ethical concerns, to better detect and prevent market manipulation in the financial market.