Data Science and Analytics Requirements:The Importance of Data Science and Analytics in a Digital Age
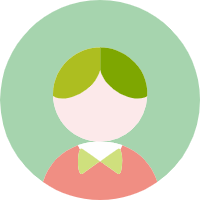
Data Science and Analytics Requirements: Addressing Challenges in Data Science and Analytics through Innovation
Data science and analytics have become essential components of modern business and technological innovation. With the exponential growth of data generated by the digital revolution, organizations are increasingly relying on data science and analytics to make informed decisions, optimize processes, and drive growth. However, the rapid advancements in data science and analytics also present several challenges that need to be addressed to fully harness their potential. This article explores the current requirements in data science and analytics, highlights the challenges faced in their implementation, and discusses innovative solutions to address these challenges.
Current Requirements in Data Science and Analytics
1. Data Availability and Quality
One of the key requirements in data science and analytics is access to high-quality, accurate, and reliable data. As data becomes more dispersed, diverse, and complex, organizations need to invest in data management and integration tools to ensure the quality of the data they work with. This includes data cleaning, data validation, and data standardization, which are crucial for the successful implementation of data science and analytics projects.
2. Data Science and Analytics Skills
The demand for data science and analytics professionals is on the rise, as organizations seek to harness the power of data to drive innovation and growth. To meet this demand, organizations need to invest in training and education programs to upskill their current workforce or recruit talent with relevant skills. This includes developing a strong foundation in data science, machine learning, and artificial intelligence, as well as training in data visualization and communication.
3. Data Security and Privacy
As data science and analytics become more prevalent, concerns about data security and privacy are increasing. Organizations need to invest in robust data security measures to protect sensitive information and comply with data protection regulations. This includes implementing robust access controls, data encryption, and security audits to prevent data breaches and unauthorized access.
4. Collaboration and Communication
Data science and analytics projects often involve multiple stakeholders, requiring effective collaboration and communication. Organizations need to establish clear communication channels and collaboration tools to ensure that all team members can effectively work together and share their expertise. This includes leveraging tools such as data visualization, natural language processing, and machine learning to facilitate better understanding and communication of complex data.
Challenges in Data Science and Analytics
1. Data Silos and Integration
One of the main challenges in data science and analytics is the existence of data silos within organizations. This can prevent the full integration and analysis of data, limiting the effectiveness of data-driven decisions. To address this challenge, organizations need to invest in data integration and management tools to ensure that all data can be accessed, analyzed, and shared across the organization.
2. Lack of Data Science and Analytics Skills
As mentioned earlier, the demand for data science and analytics professionals is increasing, but the supply of skilled talent is not keeping up. This has led to a significant talent gap, which can hinder the implementation of data science and analytics projects. Organizations need to invest in talent acquisition and retention strategies to address this challenge and ensure a steady stream of skilled professionals.
3. Complexity of Data Science and Analytics Projects
Data science and analytics projects often involve complex data sets and multiple datasets, making it challenging for organizations to effectively analyze and interpret the data. This can lead to incorrect conclusions and poor decision-making. To address this challenge, organizations need to invest in advanced data science and analytics tools and techniques, such as machine learning and artificial intelligence, to help them navigate the complexity of their data.
Innovative Solutions to Address Challenges in Data Science and Analytics
1. Data Science and Analytics Platforms
Data science and analytics platforms can help organizations overcome some of the challenges they face. These platforms provide a centralized location for data management, collaboration, and analysis, allowing teams to easily access, integrate, and analyze data from multiple sources. By adopting data science and analytics platforms, organizations can streamline their data processes and improve their data-driven decision-making.
2. Artificial Intelligence and Machine Learning
Artificial intelligence and machine learning can help organizations overcome the challenges associated with complex data sets and multiple datasets. These advanced technologies can automatically analyze and interpret data, providing valuable insights and recommendations for decision-making. By investing in artificial intelligence and machine learning, organizations can enhance their data science and analytics capabilities and drive innovation.
3. Training and Education Programs
To address the challenge of a lack of data science and analytics skills, organizations need to invest in training and education programs. This includes providing in-house training, partnering with educational institutions, and developing online courses and certifications. By upskilling their current workforce or recruiting talent with relevant skills, organizations can ensure that they have the necessary expertise to successfully implement data science and analytics projects.
Data science and analytics are critical components of modern business and technological innovation. However, the rapid advancements in data science and analytics also present several challenges that need to be addressed to fully harness their potential. By investing in innovative solutions, such as data science and analytics platforms, artificial intelligence and machine learning, and training and education programs, organizations can overcome these challenges and effectively address the requirements in data science and analytics. Ultimately, the success of data science and analytics depend on the ability of organizations to effectively harness the power of data and drive innovation through innovation.