Blockchain-based federated learning in medicine: Applications and Challenges of Blockchain Technology in Medicine
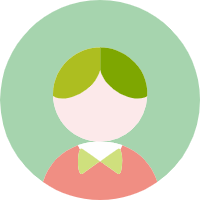
Blockchain technology has been making waves in various industries, and healthcare is no exception. The potential of blockchain in medicine is immense, with its capabilities in improving data security, transparency, and efficiency. One of the most promising applications of blockchain in medicine is federated learning, which allows medical devices and algorithms to collaborate and share knowledge without exposing sensitive data. This article aims to explore the applications of blockchain-based federated learning in medicine, as well as the challenges and implications of this technology in the healthcare sector.
Applications of Blockchain-based Federated Learning in Medicine
1. Medical Imaging and Diagnostics
One of the most promising applications of blockchain-based federated learning in medicine is medical imaging and diagnostics. By enabling secure and privacy-preserving data sharing between medical devices and algorithms, federated learning can help improve the accuracy and efficiency of medical imaging systems. This can lead to earlier detection of diseases and better patient outcomes.
2. Personalized Medicine
Blockchain-based federated learning can also play a significant role in personalized medicine. By analyzing data from various sources, such as genetic information, medical records, and wearable devices, federated learning can help develop personalized treatment plans and drug therapies. This can lead to more effective and targeted medical treatments, ultimately improving patient outcomes.
3. Drug Discovery and Development
The process of drug discovery and development is time-consuming and expensive. Blockchain-based federated learning can help streamline this process by enabling collaboration between researchers, institutions, and pharmaceutical companies. By sharing data and knowledge, federated learning can help accelerate drug discovery and development, ultimately leading to the discovery of new treatments and drugs.
Challenges and Implications of Blockchain-based Federated Learning in Medicine
1. Data Privacy and Security
One of the primary concerns with implementing blockchain-based federated learning in medicine is data privacy and security. Ensuring the security and confidentiality of sensitive medical data is crucial, as breaches can have severe consequences for patients and healthcare providers. Ensuring the robustness and security of blockchain systems is also essential to prevent unauthorized access to sensitive data.
2. Data Standardization and Integration
Another challenge in implementing blockchain-based federated learning in medicine is data standardization and integration. Different healthcare providers and institutions may use different data formats and standards, which can hinder collaboration and data sharing. Ensuring data standardization and integration is crucial for the successful implementation of blockchain-based federated learning in medicine.
3. Regulatory and Ethical Considerations
The implementation of blockchain-based federated learning in medicine also raises several regulatory and ethical concerns. Ensuring compliance with existing regulations and addressing ethical concerns, such as patient consent and privacy, is essential before implementing this technology in the healthcare sector.
Blockchain-based federated learning has the potential to revolutionize medicine by improving data security, transparency, and efficiency. However, addressing the challenges and implications of this technology is crucial for its successful implementation in the healthcare sector. By overcoming these challenges and harnessing the power of blockchain-based federated learning, medicine can embark on a new era of innovation, improving patient outcomes and transforming the way we approach healthcare.