training data for sentiment analysis: Collecting and Preparing Data for Sentiment Analysis
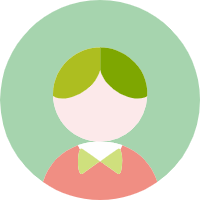
Sentiment analysis, also known as opinion mining, is the process of automating the extraction of subjective information from text data. It is a crucial component of natural language processing (NLP) and has gained significant attention in recent years, particularly with the advent of social media and online reviews. The goal of sentiment analysis is to classify text data into positive, negative, or neutral categories, allowing businesses and organizations to gauge customer sentiment and make informed decisions.
To perform sentiment analysis effectively, one must have a large and diverse dataset of text data, labeled with appropriate sentiment labels. In this article, we will discuss the important aspects of collecting and preparing data for sentiment analysis, with a focus on data quality, diversity, and label availability.
Data Collection
Data collection is the first and most critical step in the sentiment analysis process. The data should be gathered from various sources, such as social media platforms, customer reviews, online forums, and news articles. The sources should be diverse and represent different domains, such as e-commerce, healthcare, and entertainment. This will ensure that the sentiment analysis model is trained on a wide range of text data and can generalize well across different domains and contexts.
Data Quality
Data quality is another essential aspect of training a successful sentiment analysis model. The collected data should be clean, free from errors and inaccuracies. This includes removing duplicate data, correcting spelling errors, and fixing grammatical issues. Additionally, the data should be cleaned of inappropriate content, such as profanity or hate speech, to ensure that the model is trained on appropriate and meaningful data.
Data Diversity
Data diversity is crucial for training a strong sentiment analysis model. The dataset should include a wide range of text data, such as short messages, long essays, quotes, and tweets. This will ensure that the model is trained to handle various text formats and lengths, and can generalize well across different contexts.
Label Availability
For each piece of text data in the dataset, it is essential to have a corresponding sentiment label. The labels should be evenly distributed across the different sentiment categories (positive, negative, and neutral) to ensure that the model is trained on an appropriate balance of data. It is also important to have a balanced dataset, with an equal number of positive, negative, and neutral samples, to prevent the model from being biased towards a particular sentiment category.
Collecting and preparing high-quality data for sentiment analysis is crucial for training a successful and generalizable model. By focusing on data quality, diversity, and label availability, researchers and developers can create robust sentiment analysis models that can accurately interpret and predict customer sentiment across various domains and contexts. As the importance of sentiment analysis continues to grow, ensuring the quality and availability of training data will be essential in developing effective and reliable sentiment analysis solutions.