Data for Sentiment Analysis NLP: Understanding the Role of Data in Sentiment Analysis Natural Language Processing
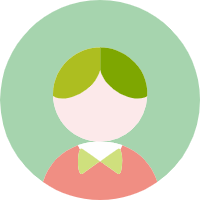
Sentiment analysis, also known as opinion mining, is a crucial aspect of natural language processing (NLP). It involves the automatic extraction of subjective information from text data, such as the opinions, emotions, and attitudes expressed by the authors. As the volume of text data generated continues to grow, the need for efficient and accurate sentiment analysis becomes increasingly important. This article aims to explore the role of data in sentiment analysis NLP, focusing on the importance of data quality, quantity, and diversity.
Data Quality
The quality of data plays a crucial role in sentiment analysis. High-quality data allows for more accurate and reliable analysis, while low-quality data can lead to inaccurate and biased results. In sentiment analysis, quality data refers to the accuracy, completeness, and consistency of the text data. Accuracy is essential as inaccurate data can lead to misinterpretation of the sentiment. Completeness refers to the full representation of the sentiment, while consistency refers to the consistency of the sentiment expressed across the data.
Data Quantity
Quantity is another essential aspect of data in sentiment analysis. The more data available, the more accurate and comprehensive the sentiment analysis can be. Large volumes of data allow for the identification of patterns and trends, which can help improve the understanding of the sentiment expressed. However, having too much data can also lead to challenges such as data overload, which can hinder the analysis process. As a result, it is crucial to strike a balance between data quantity and quality, ensuring that the data is both large enough to be useful and high enough in quality to produce accurate results.
Data Diversity
The diversity of data plays a significant role in sentiment analysis. Data diversity refers to the variety of sources, genres, and styles of text data available. This diversity allows for a more comprehensive understanding of the sentiment expressed, as it covers a range of perspectives and emotions. However, too little diversity in the data can lead to biases and limitations in the sentiment analysis. For example, if all the data comes from a specific source or genre, it may not be able to accurately represent the full range of opinions and emotions expressed in the broader population.
In conclusion, the role of data in sentiment analysis NLP is crucial, with quality, quantity, and diversity all playing important roles in the success of the analysis. Ensuring high-quality data, having sufficient quantity, and utilizing diverse data sources are essential for accurate and reliable sentiment analysis. As the volume of text data continues to grow, it is essential to understand the importance of data in sentiment analysis and to leverage its potential for improved NLP applications.